Picture 1 of 1
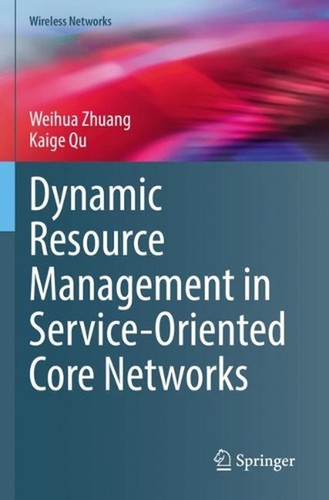
Dynamic Resource Management in Service-Orient ed Core Networks by Weihua Zhuang (
Condition:
7 available
Postage:
Located in: Fairfield, Ohio, United States
Delivery:
Estimated between Sat, 18 May and Thu, 30 May to 43230
Returns:
Coverage:
Read item description or contact seller for details. See all detailsSee all details on coverage
(Not eligible for eBay purchase protection programmes)
Shop with confidence
Seller information
- 98.2% positive feedback
Registered as a Business Seller
Seller assumes all responsibility for this listing.
eBay item number:395359911159
Item specifics
- Condition
- Brand New: A new, unread, unused book in perfect condition with no missing or damaged pages. See all condition definitionsopens in a new window or tab
- ISBN-13
- 9783030871383
- Book Title
- Dynamic Resource Management in Service-Oriented Core Networks
- ISBN
- 9783030871383
- Publication Year
- 2022
- Series
- Wireless Networks Ser.
- Type
- Textbook
- Format
- Trade Paperback
- Language
- English
- Publication Name
- Dynamic Resource Management in Service-Oriented Core Networks
- Item Length
- 9.3in
- Publisher
- Springer International Publishing A&G
- Item Width
- 6.1in
- Item Weight
- 10.4 Oz
- Number of Pages
- Xii, 173 Pages
About this product
Product Information
This book provides a timely and comprehensive study of dynamic resource management for network slicing in service-oriented fifth-generation (5G) and beyond core networks. This includes the perspective of developing efficient computation resource provisioning and scheduling solutions to guarantee consistent service performance in terms of end-to-end (E2E) data delivery delay. Network slicing is enabled by the software defined networking (SDN) and network function virtualization (NFV) paradigms. For a network slice with a target traffic load, the E2E service delivery is enabled by virtual network function (VNF) placement and traffic routing with static resource allocations. When data traffic enters the network, the traffic load is dynamic and can deviate from the target value, potentially leading to QoS performance degradation and network congestion. Data traffic has dynamics in different time granularities. For example, the traffic statistics (e.g., mean and variance) can be non-stationary and experience significant changes in a coarse time granularity, which are usually predictable. Within a long time duration with stationary traffic statistics, there are traffic dynamics in small timescales, which are usually highly bursty and unpredictable. To provide continuous QoS performance guarantee and ensure efficient and fair operation of the network slices over time, it is essential to develop dynamic resource management schemes for the embedded services in the presence of traffic dynamics during virtual network operation. Queueing theory is used in system modeling, and different techniques including optimization and machine learning are applied to solving the dynamic resource management problems. Based on a simplified M/M/1 queueing model with Poisson traffic arrivals, an optimization model for flow migration is presented to accommodate the large-timescale changes in the average traffic rates with average E2E delay guarantee, while addressing a trade-off between load balancing and flow migration overhead. To overcome the limitations of Poisson traffic model, the authors present a machine learning approach for dynamic VNF resource scaling and migration. The new solution captures the inherent traffic patterns in a real-world traffic trace with non-stationary traffic statistics in large timescale, predicts resource demands for VNF resource scaling, and triggers adaptive VNF migration decision making, to achieve load balancing, migration cost reduction, and resource overloading penalty suppression in the long run. Both supervised and unsupervised machine learning tools are investigated for dynamic resource management. To accommodate the traffic dynamics in small time granularities, the authors present a dynamic VNF scheduling scheme to coordinate the scheduling among VNFs of multiple services, which achieves network utility maximization with delay guarantee for each service. Researchers and graduate students working in the areas of electrical engineering, computing engineering and computer science will find this book useful as a reference or secondary text. Professionals in industry seeking solutions to dynamic resource management for 5G and beyond networks will also want to purchase this book.
Product Identifiers
Publisher
Springer International Publishing A&G
ISBN-10
303087138x
ISBN-13
9783030871383
eBay Product ID (ePID)
16058363068
Product Key Features
Publication Name
Dynamic Resource Management in Service-Oriented Core Networks
Format
Trade Paperback
Language
English
Publication Year
2022
Series
Wireless Networks Ser.
Type
Textbook
Number of Pages
Xii, 173 Pages
Dimensions
Item Length
9.3in
Item Width
6.1in
Item Weight
10.4 Oz
Additional Product Features
Number of Volumes
1 Vol.
Lc Classification Number
Tk5105.5-5105.9
Table of Content
Chapter 1 Introduction -. 1.1 Service-Oriented Core Networks-. 1.1.1 Software-Defined Networking (SDN)-. 1.1.2 Network Function Virtualization (NFV)-. 1.1.3 Service Function Chaining-. 1.2 Network Slicing Framework-. 1.2.1 Infrastructure Domain-. 1.2.2 Tenant Domain-. 1.2.3 SDN-NFV Integration-. 1.3 Multi-Timescale Dynamic Resource Management-. 1.3.1 Multi-Timescale Core Network Traffic Dynamics-. 1.3.2 Dynamic Resource Provisioning in Large Timescale-. 1.3.3 Dynamic Resource Scheduling in Small Timescale-. 1.4 Research Contributions-. 1.5 Outline-. References-. Chapter 2 System Model -. 2.1 Services-. 2.2 Virtual Resource Pool-. 2.3 Placement and Scheduling of Virtual Network Function (VNF)-. 2.3 Migration Cost and Reconfiguration Overhead-. References-. Chapter 3 Dynamic Flow Migration: A Model-Based Optimization Approach -. 3.1 Model Assumptions-. 3.1.1 M/M/1 VNF Packet Processing Queueing Model-. 3.1.2 Generalized Processor Sharing (GPS)-. 3.2 Optimization Model for Dynamic Flow Migration-. 3.3 Mixed Integer Quadratically Constrained Programming (MIQCP) Problem Transformation-. 3.3.1 Optimality Gap-. 3.3.2 Optimal Solution Mapping-. 3.4 Low-Complexity Heuristic Flow Migration Algorithm-. 3.4.1 Algorithm Overview-. 3.4.2 Redistribution of Hop Delay Bounds-. 3.4.3 Migration Decision-. 3.4.4 Iterative Resource Loading Threshold Update-. 3.4.5 Complexity Analysis-. 3.5 Simulation Results-. 3.6 Summary-. References-. Chapter 4 Dynamic VNF Resource Scaling and Migration: A Machine Learning Approach -. 4.1 Nonstationary Traffic Model-. 4.2 Machine Learning Tools for Analysis and Decision-. 4.2.1 Bayesian Conjugate Analysis-. 4.2.2 Gaussian Process Regression-. 4.2.3 Reinforcement Learning-. 4.3 Resource Demand Prediction for Dynamic VNF Resource Scaling-. 4.3.1 Bayesian Online Change Point Detection-. 4.3.2 Traffic Parameter Learning-. 4.3.3 Resource Demand Prediction-. 4.4 Deep Reinforcement Learning for Dynamic VNF Migration-. 4.4.1 MarkovDecision Process-. 4.4.2 Penalty-Aware Deep Q-Learning Algorithm-. 4.5 Simulation Results-. 4.6 Summary-. References-. Chapter 5 Dynamic VNF Scheduling for Network Utility Maximization -. 5.1 Discrete-Time VNF Packet Processing Queueing Model-. 5.1.1 Physical Packet Processing Queue-. 5.1.2 Delay-Aware Virtual Packet Processing Queue-. 5.2 Stochastic VNF Scheduling: Problem and Solution-. 5.2.1 Stochastic Problem Formulation-. 5.2.2 Lyapunov Optimization and Problem Transformation-. 5.2.3 Online Distributed Algorithm-. 5.3 VNF Scheduling with Packet Rushing-. 5.3.1 Packet Rushing Analysis-. 5.3.2 Modified VNF Scheduling Algorithm-. 5.4 Simulation Results-. 5.5 Summary-. References-. Chapter 6 Conclusions and Future Research Directions -. 6.1 Conclusions-. 6.2 Future Research Directions-. References.
Copyright Date
2021
Topic
Mobile & Wireless Communications, Probability & Statistics / General, Networking / General
Illustrated
Yes
Genre
Computers, Technology & Engineering, Mathematics
Item description from the seller
Seller assumes all responsibility for this listing.
eBay item number:395359911159
Postage and handling
Item location:
Fairfield, Ohio, United States
Post to:
Afghanistan, Albania, Algeria, Andorra, Angola, Anguilla, Antigua and Barbuda, Argentina, Armenia, Aruba, Australia, Austria, Azerbaijan Republic, Bahamas, Bahrain, Bangladesh, Belgium, Belize, Benin, Bermuda, Bhutan, Bolivia, Bosnia and Herzegovina, Botswana, Brazil, British Virgin Islands, Brunei Darussalam, Bulgaria, Burkina Faso, Burundi, Cambodia, Cameroon, Canada, Cape Verde Islands, Cayman Islands, Central African Republic, Chad, Chile, China, Colombia, Comoros, Cook Islands, Costa Rica, Cyprus, Czech Republic, Côte d'Ivoire (Ivory Coast), Democratic Republic of the Congo, Denmark, Djibouti, Dominica, Dominican Republic, Ecuador, Egypt, El Salvador, Equatorial Guinea, Eritrea, Estonia, Ethiopia, Falkland Islands (Islas Malvinas), Fiji, Finland, France, Gabon Republic, Gambia, Georgia, Germany, Ghana, Gibraltar, Greece, Greenland, Grenada, Guatemala, Guernsey, Guinea, Guinea-Bissau, Guyana, Haiti, Honduras, Hong Kong, Hungary, Iceland, India, Indonesia, Iraq, Ireland, Israel, Italy, Jamaica, Japan, Jersey, Jordan, Kazakhstan, Kenya, Kiribati, Kuwait, Kyrgyzstan, Laos, Latvia, Lebanon, Lesotho, Liberia, Liechtenstein, Lithuania, Luxembourg, Macau, Macedonia, Madagascar, Malawi, Malaysia, Maldives, Mali, Malta, Mauritania, Mauritius, Mayotte, Mexico, Moldova, Monaco, Mongolia, Montenegro, Montserrat, Morocco, Mozambique, Namibia, Nauru, Nepal, Netherlands, Netherlands Antilles, New Zealand, Nicaragua, Niger, Nigeria, Niue, Norway, Oman, Pakistan, Paraguay, Peru, Philippines, Poland, Portugal, Qatar, Republic of Croatia, Republic of the Congo, Romania, Rwanda, Saint Helena, Saint Kitts-Nevis, Saint Lucia, Saint Pierre and Miquelon, Saint Vincent and the Grenadines, San Marino, Saudi Arabia, Senegal, Serbia, Seychelles, Sierra Leone, Singapore, Slovakia, Slovenia, Solomon Islands, Somalia, South Africa, South Korea, Spain, Sri Lanka, Suriname, Svalbard and Jan Mayen, Swaziland, Sweden, Switzerland, Taiwan, Tajikistan, Tanzania, Thailand, Togo, Tonga, Trinidad and Tobago, Tunisia, Turkey, Turkmenistan, Turks and Caicos Islands, Tuvalu, Uganda, United Arab Emirates, United Kingdom, Uruguay, Uzbekistan, Vanuatu, Vatican City State, Vietnam, Wallis and Futuna, Western Sahara, Worldwide, Yemen, Zambia, Zimbabwe
Excludes:
APO/FPO, Alaska/Hawaii, Barbados, Belarus, French Guiana, French Polynesia, Guadeloupe, Libya, Martinique, New Caledonia, Panama, Papua New Guinea, Reunion, Russian Federation, US Protectorates, Ukraine, Venezuela, Western Samoa
Postage and handling | Each additional item | To | Service | Delivery*See Delivery notes |
---|---|---|---|---|
Free postage | Free | United States | Economy Shipping | Estimated between Sat, 18 May and Thu, 30 May to 43230 |
Handling time |
---|
Will usually post within 10 business days of receiving cleared payment. |
Taxes |
---|
Taxes may be applicable at checkout. Learn moreLearn more about paying tax on eBay purchases. |
Sales Tax for an item #395359911159
Sales Tax for an item #395359911159
Seller collects sales tax for items shipped to the following states:
State | Sales Tax Rate |
---|
Return policy
After receiving the item, contact seller within | Refund will be given as |
---|---|
30 days after the buyer receives it | Money Back |
The buyer is responsible for return postage costs.
Seller feedback (1,022,049)
d***l (549)- Feedback left by buyer.
Past month
Verified purchase
A+
i***i (706)- Feedback left by buyer.
Past month
Verified purchase
Smooth Transaction
0***n (828)- Feedback left by buyer.
Past month
Verified purchase
Pleased would use this seller again