Picture 1 of 1
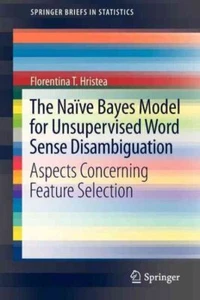
Picture 1 of 1
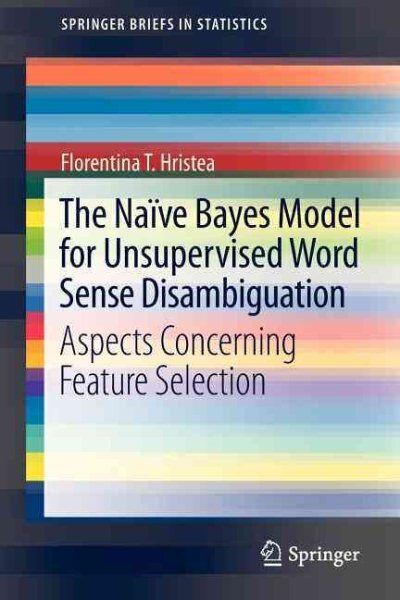
Naive Bayes Model for Unsupervised Word Sense Disambiguation : Aspects Concer...
US $51.00
ApproximatelyS$ 65.96
Condition:
Like New
A book in excellent condition. Cover is shiny and undamaged, and the dust jacket is included for hard covers. No missing or damaged pages, no creases or tears, and no underlining/highlighting of text or writing in the margins. May be very minimal identifying marks on the inside cover. Very minimal wear and tear.
2 available
Postage:
Free Economy Shipping.
Located in: Jessup, Maryland, United States
Delivery:
Estimated between Fri, 4 Oct and Thu, 10 Oct to 43230
Returns:
14 days return. Buyer pays for return shipping.
Coverage:
Read item description or contact seller for details. See all detailsSee all details on coverage
(Not eligible for eBay purchase protection programmes)
Seller assumes all responsibility for this listing.
eBay item number:364570934418
Item specifics
- Condition
- Book Title
- Naive Bayes Model for Unsupervised Word Sense Disambiguation : As
- ISBN
- 9783642336928
- Subject Area
- Mathematics, Computers, Language Arts & Disciplines
- Publication Name
- Naïve Bayes Model for Unsupervised Word Sense Disambiguation : Aspects Concerning Feature Selection
- Publisher
- Springer Berlin / Heidelberg
- Item Length
- 9.3 in
- Subject
- Probability & Statistics / General, Intelligence (Ai) & Semantics, Natural Language Processing, General, Linguistics / General
- Publication Year
- 2012
- Series
- Springerbriefs in Statistics Ser.
- Type
- Textbook
- Format
- Trade Paperback
- Language
- English
- Item Weight
- 49.9 Oz
- Item Width
- 6.1 in
- Number of Pages
- Xiii, 70 Pages
About this product
Product Identifiers
Publisher
Springer Berlin / Heidelberg
ISBN-10
3642336922
ISBN-13
9783642336928
eBay Product ID (ePID)
144070317
Product Key Features
Number of Pages
Xiii, 70 Pages
Language
English
Publication Name
Naïve Bayes Model for Unsupervised Word Sense Disambiguation : Aspects Concerning Feature Selection
Subject
Probability & Statistics / General, Intelligence (Ai) & Semantics, Natural Language Processing, General, Linguistics / General
Publication Year
2012
Type
Textbook
Subject Area
Mathematics, Computers, Language Arts & Disciplines
Series
Springerbriefs in Statistics Ser.
Format
Trade Paperback
Dimensions
Item Weight
49.9 Oz
Item Length
9.3 in
Item Width
6.1 in
Additional Product Features
Intended Audience
Scholarly & Professional
Dewey Edition
23
TitleLeading
The
Number of Volumes
1 vol.
Illustrated
Yes
Dewey Decimal
401.430285
Table Of Content
1.Preliminaries.- 2.The Naïve Bayes Model in the Context of Word Sense Disambiguation.- 3.Semantic WordNet-based Feature Selection.- 4.Syntactic Dependency-based Feature Selection.- 5.N-Gram Features for Unsupervised WSD with an Underlying Naïve Bayes Model References.- Index.
Synopsis
This book presents recent advances (from 2008 to 2012) concerning use of the Naïve Bayes model in unsupervised word sense disambiguation (WSD). While WSD, in general, has a number of important applications in various fields of artificial intelligence (information retrieval, text processing, machine translation, message understanding, man-machine communication etc.), unsupervised WSD is considered important because it is language-independent and does not require previously annotated corpora. The Naïve Bayes model has been widely used in supervised WSD, but its use in unsupervised WSD has led to more modest disambiguation results and has been less frequent. It seems that the potential of this statistical model with respect to unsupervised WSD continues to remain insufficiently explored. The present book contends that the Naïve Bayes model needs to be fed knowledge in order to perform well as a clustering technique for unsupervised WSD and examines three entirely different sources of such knowledge for feature selection: WordNet, dependency relations and web N-grams. WSD with an underlying Naïve Bayes model is ultimately positioned on the border between unsupervised and knowledge-based techniques. The benefits of feeding knowledge (of various natures) to a knowledge-lean algorithm for unsupervised WSD that uses the Naïve Bayes model as clustering technique are clearly highlighted. The discussion shows that the Naïve Bayes model still holds promise for the open problem of unsupervised WSD., This book presents recent advances (from 2008 to 2012) concerning use of the Na ve Bayes model in unsupervised word sense disambiguation (WSD). While WSD, in general, has a number of important applications in various fields of artificial intelligence (information retrieval, text processing, machine translation, message understanding, man-machine communication etc.), unsupervised WSD is considered important because it is language-independent and does not require previously annotated corpora. The Na ve Bayes model has been widely used in supervised WSD, but its use in unsupervised WSD has led to more modest disambiguation results and has been less frequent. It seems that the potential of this statistical model with respect to unsupervised WSD continues to remain insufficiently explored. The present book contends that the Na ve Bayes model needs to be fed knowledge in order to perform well as a clustering technique for unsupervised WSD and examines three entirely different sources of such knowledge for feature selection: WordNet, dependency relations and web N-grams. WSD with an underlying Na ve Bayes model is ultimately positioned on the border between unsupervised and knowledge-based techniques. The benefits of feeding knowledge (of various natures) to a knowledge-lean algorithm for unsupervised WSD that uses the Na ve Bayes model as clustering technique are clearly highlighted. The discussion shows that the Na ve Bayes model still holds promise for the open problem of unsupervised WSD., 1.Preliminaries.- 2.The Naïve Bayes Model in the Context of Word Sense Disambiguation.- 3.Semantic WordNet-based Feature Selection.- 4.Syntactic Dependency-based Feature Selection.- 5.N-Gram Features for Unsupervised WSD with an Underlying Naïve Bayes Model References.- Index.
LC Classification Number
QA276-280
Item description from the seller
Seller feedback (353,342)
- g***r (431)- Feedback left by buyer.Past monthVerified purchaseThank you!!!
- 1***d (1454)- Feedback left by buyer.Past monthVerified purchaseNice item as described, carefully packaged, fast shipping. A++ seller. Thanks!
- m***m (4415)- Feedback left by buyer.Past monthVerified purchaseExcellent Seller! Shipped quickly and was well packaged! Recommended Seller- A+++